Identifying collisionless shock crossings in data sent from spacecraft has so far been done manually or using basic algorithms. It is a tedious job that shock physicists have to go through if they want to conduct case studies or perform statistical studies. We use a machine learning approach to automatically identify shock crossings from the Magnetospheric Multiscale (MMS) spacecraft. We compiled a database of 2,797 shock crossings, spanning a period from October 2015 to December 2020, including various spacecraft-related and shock-related parameters for each event. Furthermore, we show that the shock crossings in the database are spread out in space, from the subsolar point to the far flanks. On top of that, we show that they cover a wide range of parameter space. We also present a possible scientific application of the database by looking for correlations between ion acceleration efficiency at shocks with different shock parameters, such as the angle between the upstream magnetic field and the shock normal θBn and the Alfvénic Mach number MA. We find no clear correlation between the acceleration efficiency and MA; however, we find that quasi-parallel shocks are more efficient at accelerating ions than quasi-perpendicular shocks.
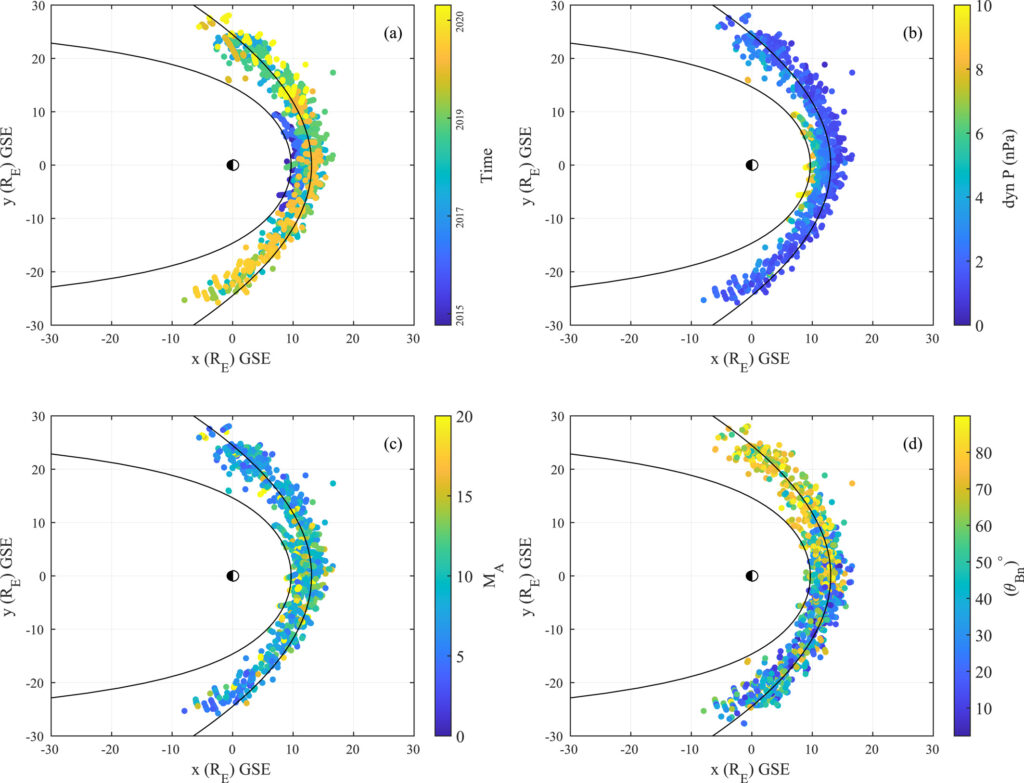
Full Article:
Lalti, A. (SHARP), Khotyaintsev, Yu. V. (SHARP), Dimmock, A. P. (SHARP), Johlander, A. (SHARP), Graham, D. B. (SHARP), Olshevsky, V. (2022). A database of MMS bow shock crossings compiled using machine learning. Journal of Geophysical Research: Space Physics, 127, doi: 10.1029/2022JA030454
License: CC BY 4.0